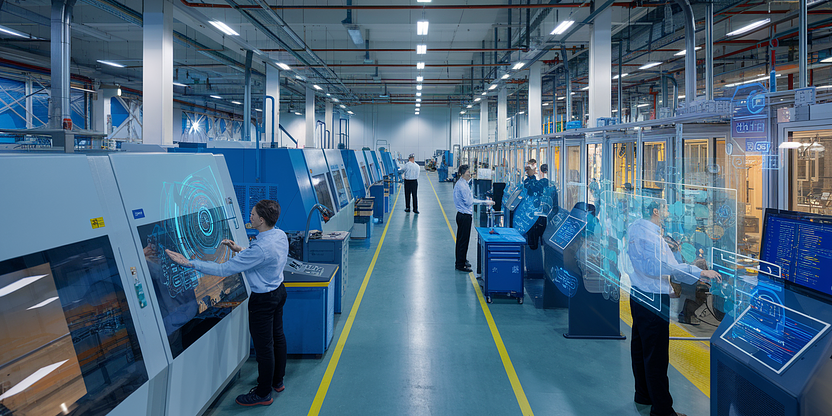
The Complete Checklist for Data Analytics in Manufacturing
Harnessing Data Analytics for Operational Excellence in Manufacturing
In today's fast-paced industrial landscape, data serves as the essential cornerstone for informed decision-making. For the manufacturing sector, where efficiency, precision, and lean operations are pivotal, data analytics offers lucrative opportunities to optimize processes, improve product quality, and reduce operational costs. By tapping into the wealth of information available, manufacturers can transform their operations and maintain a competitive edge in the marketplace.
Why Data Analytics is Essential in Manufacturing
The integration of data analytics into manufacturing is not merely a trend but a necessity. It involves converting massive volumes of raw data collected from various facets of the manufacturing process into actionable insights. These insights can enhance understanding across the production lifecycle—from supply chain management to production automation and quality control.
Improved Operational Efficiency: By analyzing data, manufacturers can identify bottlenecks in production lines, thus allowing for process improvement and resource optimization.
Predictive Maintenance: Data collected from machinery sensors can be analyzed to predict when equipment will fail or require maintenance, reducing downtime and maintenance costs.
Quality Assurance: Real-time analytics assists in ensuring that products maintain high standards of quality which reduces waste and rework.
Supply Chain Management: Data analytics helps in forecasting demand more accurately, leading to efficient inventory management and reduced logistical costs.
Cost Reduction: Streamlined processes and predictive maintenance lead to overall cost reductions.
The Analytical Process in Manufacturing
To generate value from analytics, manufacturing companies must adopt a structured and holistic approach:
Data Collection: Start with collecting data from multiple sources, including IoT devices, production logs, sensors on equipment, and enterprise systems.
Data Integration and Cleansing: Ensure that the data is accurate, relevant, and integrated from various sources to form a cohesive data pool.
Data Analysis and Modeling: Use statistical models and machine learning tools to analyze data. These analyses can focus on specific objectives such as reducing waste or improving production schedules.
Visualization and Reporting: Translate data findings into clear and actionable visual reports which facilitate quick comprehension and decision-making.
Implementation of Insights: Apply the insights gained to make adjustments or enhancements in the manufacturing process.
Continuous Monitoring and Improvement: Set up continuous feedback loops to monitor the impact of implemented changes and keep improving the processes.
Building a Robust Data Strategy
A tailored data strategy is critical to the success of data analytics in manufacturing. The steps below offer a roadmap for developing a potent data strategy:
- Evaluate your existing data capabilities and identify gaps.
- Define clear objectives aligned with your organizational goals.
- Select the appropriate tools and technologies tailored to your needs.
- Ensure data privacy and security protocols are in place.
- Foster a data-driven culture within the organization by training employees.
The Complete Checklist for Data Analytics in Manufacturing
To streamline the implementation and ongoing management of data analytics in manufacturing, use this comprehensive checklist:
- [ ] Align data analytics objectives with business goals.
- [ ] Secure buy-in from key stakeholders and build a cross-functional team.
- [ ] Inventory all data sources and identify data gaps.
- [ ] Develop a robust data governance framework.
- [ ] Ensure data quality and integrity through cleaning and integration processes.
- [ ] Select and deploy suitable analytics tools and technologies.
- [ ] Implement predictive analytics for maintenance and quality improvements.
- [ ] Establish real-time monitoring and reporting systems.
- [ ] Foster a culture of continuous improvement and training related to data analytics.
- [ ] Periodically review and adapt the data strategy to accommodate technological advances and shift in business needs.
Real-World Implementations
Several global manufacturing leaders have successfully utilized data analytics to catalyze their performance and innovation:
General Electric (GE): GE's Predix platform utilizes data analytics to enhance the efficiency and predictability of its solutions in manufacturing, from reducing manufacturing cycle times to improving product designs through iterative analysis.
Toyota: Known for its efficiency, Toyota leverages real-time data analytics to maintain its just-in-time (JIT) inventory system, ensuring optimal inventory management and production scheduling.
Siemens: Siemens employs advanced data analytics across its manufacturing operations to predictively maintain equipment, prevent downtime, and improve production quality through a robust Industrial IoT platform.
Conclusion
Incorporating data analytics into manufacturing not only optimizes production processes but also propels strategic decision-making. Forward-thinking manufacturers that invest in robust data analytics frameworks stand to gain significant competitive advantages. By adopting comprehensive implementation strategies and leveraging advanced analytics tools, companies can achieve new levels of efficiency, product quality, and innovation. The future of manufacturing is decidedly data-driven, and those who embrace this transformation will thrive in an increasingly complex global market.